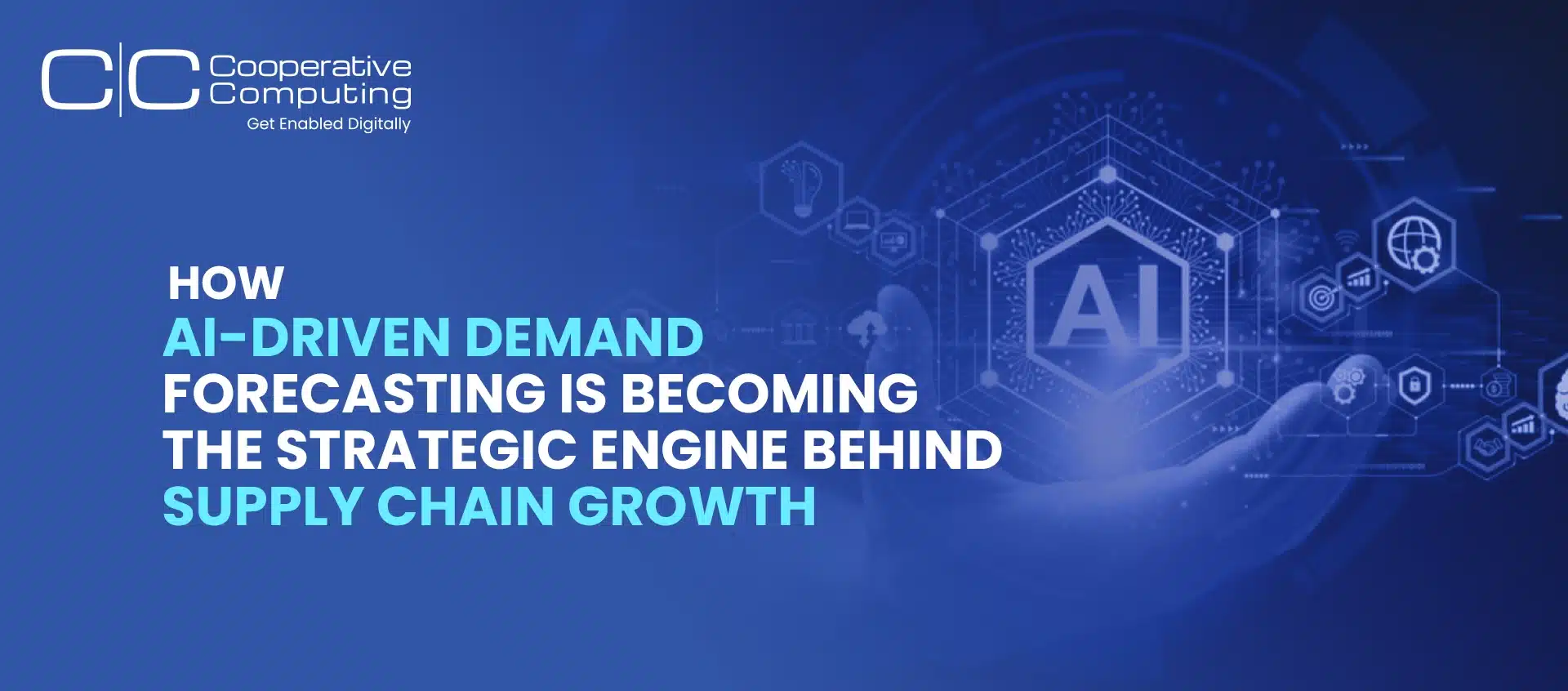
How AI-Driven Demand Forecasting Is Becoming the Strategic Engine Behind Supply Chain Growth
In today’s hyperconnected, automated economy, the pressure to anticipate, adapt, and deliver has escalated dramatically. From climate volatility to consumer unpredictability, supply chain leaders are navigating a business landscape shaped by constant disruption. The ability to forecast demand accurately is no longer confined to supply chain teams, it’s now a matter of enterprise performance.
Across boardrooms, demand forecasting has moved up the strategic agenda. Growth-minded CEOs now understand that resilience and responsiveness hinge on the ability to predict what customers will need, when, and where. With conventional models losing traction amid fragmented channels and increasingly erratic signals, attention is turning to more intelligent solutions.
At the center of this shift is AI demand forecasting, a discipline that enables companies to detect subtle market patterns, correct course faster, and align operations in real time. It’s redefining how modern supply chains compete: not by reacting to the past, but by anticipating what’s next with precision and speed.
What’s Broken with Traditional Forecasting Models
For years, demand planning has relied on backward-looking spreadsheets, static ERP inputs, and forecasting methods anchored in historical trends. These systems were built for stable markets, not dynamic ecosystems shaped by unpredictable consumer behaviors, promotional spikes, and real-time demand signals.
In the current environment, traditional models can’t process the sheer volume and velocity of data across digital and physical channels. Forecasting errors are common; many organizations still operate with high error rates, which directly affect inventory, cash flow, and customer satisfaction.
-
- Excess inventory leads to locked-up capital and forced markdowns
-
- Understocking results in missed revenue and damaged brand trust
-
- Emergency sourcing adds cost, while reactive decisions increase operational stress
-
- Siloed planning between departments slows down response times
Perhaps most concerning is the organizational behavior that these inaccuracies drive: teams lose trust in forecasts and begin second-guessing the system, leading to duplicated efforts and inflated buffers. Instead of focusing on performance, leadership is left to manage risk with incomplete visibility.
This erosion of confidence is more than a supply chain issue; it limits strategic decision-making at every level. That’s why forward-looking organizations are pivoting toward intelligent demand planning backed by AI, where forecasts are dynamic, context-aware, and continuously improving.
How AI Demand Forecasting Works
Unlike traditional forecasting tools that apply static formulas to yesterday’s data, AI demand forecasting creates a dynamic decision-making layer, drawing on vast, multidimensional datasets and adapting in real time to market shifts. This intelligence framework fundamentally transforms how organizations plan and operate.
1. Integrated Data Ingestion
At its core, AI-driven forecasting depends on data depth and diversity. The system ingests inputs from:
-
- Historical sales, POS systems, and fulfillment data
-
- Marketing calendars and promotional events
-
- External signals such as weather, seasonality, and macroeconomic trends
-
- Real-time demand behavior across web, mobile, and in-store channels
By connecting these signals across functions, AI provides a holistic view of demand drivers, enabling forecasts to reflect current reality rather than outdated assumptions.
2. Machine Learning-Based Modeling
With rich datasets in place, AI models apply advanced algorithms to detect non-obvious patterns and relationships. These models are trained to account for:
-
- Seasonal variability and promotional lift
-
- Product interdependencies (e.g., halo and cannibalization effects)
-
- Regional and channel-specific trends
-
- Demand anomalies caused by global or local events
Instead of relying on fixed rules, AI evolves with every data point, ensuring that models remain relevant and increasingly accurate over time.
3. Predictive Output Generation
The result of this modeling is high-resolution, forward-looking intelligence that includes:
-
- Accurate demand curves at SKU, region, and time level granularity
-
- Inventory triggers aligned with lead times and fulfillment constraints
-
- Strategic alerts for procurement, pricing, and merchandising teams
-
- Planning inputs for logistics and supplier orchestration
These outputs aren’t just estimates, they’re decision enablers that feed directly into broader supply chain, finance, and sales operations.
4. Continuous Learning Through Closed-Loop Feedback
Where AI truly distinguishes itself is in its ability to learn from execution outcomes. As real-world data flows back, actual orders, cancellations, returns, or sell-through, forecasting models are recalibrated automatically. This closed-loop learning ensures continuous improvement and reduces forecast bias, making planning more resilient over time.
This intelligence layer supports more than just better numbers, it empowers proactive scenario planning, smarter capacity utilization, and aligned decision-making across the enterprise. In essence, predictive analytics in operations becomes the strategic bridge between demand sensing and fulfillment execution.
From Cost Center to Growth Engine
The narrative around supply chain planning has long been defined by cost containment, reducing waste, improving efficiency, and optimizing spend. But the emergence of AI demand forecasting is shifting this perception. Intelligent planning is no longer just a control function, it is now a critical driver of growth, margin improvement, and market agility.
Inventory Optimization with Precision
AI enables organizations to forecast demand more granularly and accurately across products, regions, and time horizons. This granularity leads to a measurable reduction in safety stock and excess inventory, often between 20% and 30%. By minimizing overstock without risking availability, businesses free up working capital and create leaner, more responsive networks.
Higher Service Levels, Lower Volatility
Smarter forecasting drives better availability. When planning aligns with actual demand patterns, customers get the right products, at the right place, at the right time. That translates to improved fill rates, fewer stockouts, and stronger brand trust, all without bloating the system with buffer stock.
Margin Protection and Reduced Disruption Costs
Emergency procurement and expedited fulfillment come at a premium. By predicting demand spikes and variability more accurately, organizations can secure supply proactively, avoiding margin erosion due to last-minute sourcing or reactive logistics.
Smarter Fulfillment Allocation
AI allows for dynamic network orchestration, where demand is mapped to fulfillment capabilities in real time. This means faster delivery, reduced transportation costs, and improved warehouse utilization, all contributing to improved profitability.
At this level, AI in supply chain management transcends operational efficiency. It becomes a strategic lever for profitable growth, activating revenue, protecting margin, and enabling competitive agility.
Cross-Industry Proof Points
The value of intelligent demand planning is no longer theoretical; it’s already being realized by category leaders across industries. From global retailers to complex manufacturing networks, organizations adopting AI-powered forecasting are posting tangible performance improvements.
Retail: Driving Store-Level Precision and Margin Efficiency
Leading retail chains are integrating AI demand forecasting into their store-level planning systems, allowing them to adjust product assortment and replenishment dynamically based on real-time sales trends, promotions, and footfall data. The result? Notable reductions in excess inventory and marked improvement in in-stock rates without over-ordering.
Manufacturing: Synchronizing Demand with Production Capacity
In discrete manufacturing, AI has enabled more accurate long-horizon forecasting by incorporating factors such as global order flows, commodity price trends, and even geopolitical risk. This alignment between market signals and production planning has reduced backorders, smoothed plant loads, and improved OTIF (on-time, in-full) metrics substantially.
CPG and Distribution: Unlocking Scalability Through Visibility
Fast-moving consumer goods companies have embedded AI into their planning stack to improve forecast granularity at the SKU and location level. With predictive insights, they are reducing cost-to-serve and automating replenishment cycles, driving improved supply chain reliability even during demand surges.
Executive Takeaway
These organizations aren’t simply forecasting better, they’re planning smarter, executing faster, and scaling with confidence. The early adopters of predictive analytics in operations are building a widening moat, reshaping their industries by treating intelligence not as a tool, but as a foundation.
Building the Intelligent Forecasting Engine
While the benefits of AI-driven forecasting are clear, implementation at enterprise scale requires strategic precision. For AI demand forecasting to move beyond proof of concept and deliver transformative value, companies must get three foundational pillars right.
1. Unified, Clean, Connected Data
AI cannot function in fragmented systems. Forecasting engines need access to integrated datasets from ERP, WMS, CRM, POS, and third-party sources. Without a unified data foundation, even the most advanced models produce limited value. Clean, structured, and continuously refreshed data is the core of forecast accuracy.
2. Cross-Functional Alignment and Shared Ownership
Planning is no longer a siloed function. Demand forecasts impact procurement, sales, finance, and operations. Organizations must align stakeholders around shared KPIs and ensure that planning insights are embedded into cross-functional decision-making. The shift to AI forecasting must be seen as an enterprise enablement, not a tech project.
3. Real-Time Visibility and Adaptive Feedback Loops
AI systems thrive on continuous learning. By embedding real-time monitoring and feedback mechanisms, enterprises ensure that forecasting remains adaptive. Variances between forecast and actuals should automatically retrain the model, enabling ongoing precision and agility in execution.
4. Strategic Enablement Partners
Implementing AI in supply chain management at scale requires more than tools; it requires the right expertise. Technology enablement partners play a critical role in accelerating design, implementation, and adoption while tailoring solutions to industry-specific complexity.
The transition to intelligent demand planning is not simply a digital upgrade, it’s a re-architecture of how planning, forecasting, and decision-making interact. When done right, the payoff is not just operational, it’s strategic, scalable, and sustained.
Forecasting as a Boardroom Metric
In 2025, demand forecasting has evolved far beyond its traditional role as a functional tool within supply chain departments. It is now recognized as a core input in enterprise performance management, influencing strategic decisions across the C-suite. For CEOs, CFOs, and COOs, the quality of forecasting is no longer just a supply chain concern, it’s a direct reflection of the organization’s agility, cost structure, and readiness for growth.
Forecast accuracy has become a proxy for enterprise intelligence. It shapes how confidently executives can make capital allocation decisions, manage liquidity, plan workforce needs, and respond to competitive shifts. A missed forecast no longer just impacts logistics; it reverberates across pricing, procurement, customer experience, and ultimately, shareholder value.
-
- For CEOs, accurate demand signals enable market-responsive growth strategies while mitigating the risk of overextension or underinvestment.
-
- For CFOs, forecasting informs cash flow planning, working capital optimization, and cost-of-goods predictability; areas that directly impact profitability and margin management.
-
- For COOs, demand precision allows for proactive resource allocation across manufacturing, fulfillment, and last-mile delivery, leading to more resilient and scalable operations.
This is why AI demand forecasting has moved from operational dashboards to boardroom scorecards. Enterprises can no longer afford planning cycles that lag behind the market or rest on outdated models. By adopting predictive analytics in operations, leaders can shift forecasting from reactive estimation to real-time orchestration, where strategic decisions are continuously informed by dynamic, AI-powered insights.
Elevating forecasting to this level also demands a shift in mindset: from viewing demand planning as a tactical function to recognizing it as a strategic enabler of long-term competitiveness. It becomes not just a question of what will be sold, but how the enterprise will shape and fulfill that demand profitably, sustainably, and at scale.
Ready to turn AI into action? Download our executive AI Use Cases Deck to see how industry leaders are driving forecasting precision, operational agility, and measurable ROI with intelligent planning.
When Intelligence Leads, Operations Follow with Confidence
The transformation is underway. Organizations are re-architecting how they sense demand, allocate resources, and respond to market conditions, not with guesswork, but with intelligence. As supply chains grow more complex and customer expectations escalate, reacting late is no longer an option. Precision must be built in from the start.
AI demand forecasting is no longer a frontier technology. It has become the operational infrastructure that underpins modern supply chain performance. The companies embedding it today are not just improving efficiency; they are strengthening resilience, reducing volatility, and gaining decision velocity in markets that reward speed and accuracy.
This shift also marks a broader redefinition of competitiveness. Growth will not go to those with more data, but to those with better foresight, those who can translate data into timely, actionable decisions that scale. That’s where intelligent demand planning becomes not just valuable, but indispensable.
At Cooperative Computing, we help enterprises make that shift, from static forecasting to adaptive, AI-enabled planning ecosystems. Through our digital enablement framework, we align data, systems, and stakeholders to deliver real-time insights and lasting impact.
Let’s redefine what forecasting means for your business. Partner with us to embed predictive intelligence at the core of your supply chain strategy.
Explore More
Elevate your operational efficiency using the latest technologies and strategic insights. Dive into our technical guide tailored for business leaders.
IHS Markit predicts that the bottlenecks in the supply chain that have plagued this year will persist until 2022 and even 2023. The logistics dilemma is expected to worsen due to economic recovery, labor shortages, and overworked transportation infrastructure. The Adverse Effects Of Supply Chain Delays The extension of supply chain problems is to blame […]
In a world driven by rapid technological advancement and shifting market dynamics, businesses are under constant pressure to adapt and grow while maintaining operational efficiency. Seamless operations are a necessity for businesses aiming to stay competitive and relevant in today’s fast-paced environment. At the heart of this efficiency lies scalable infrastructure. More than a technical […]